FEATURED
Is decentralization important for AI?
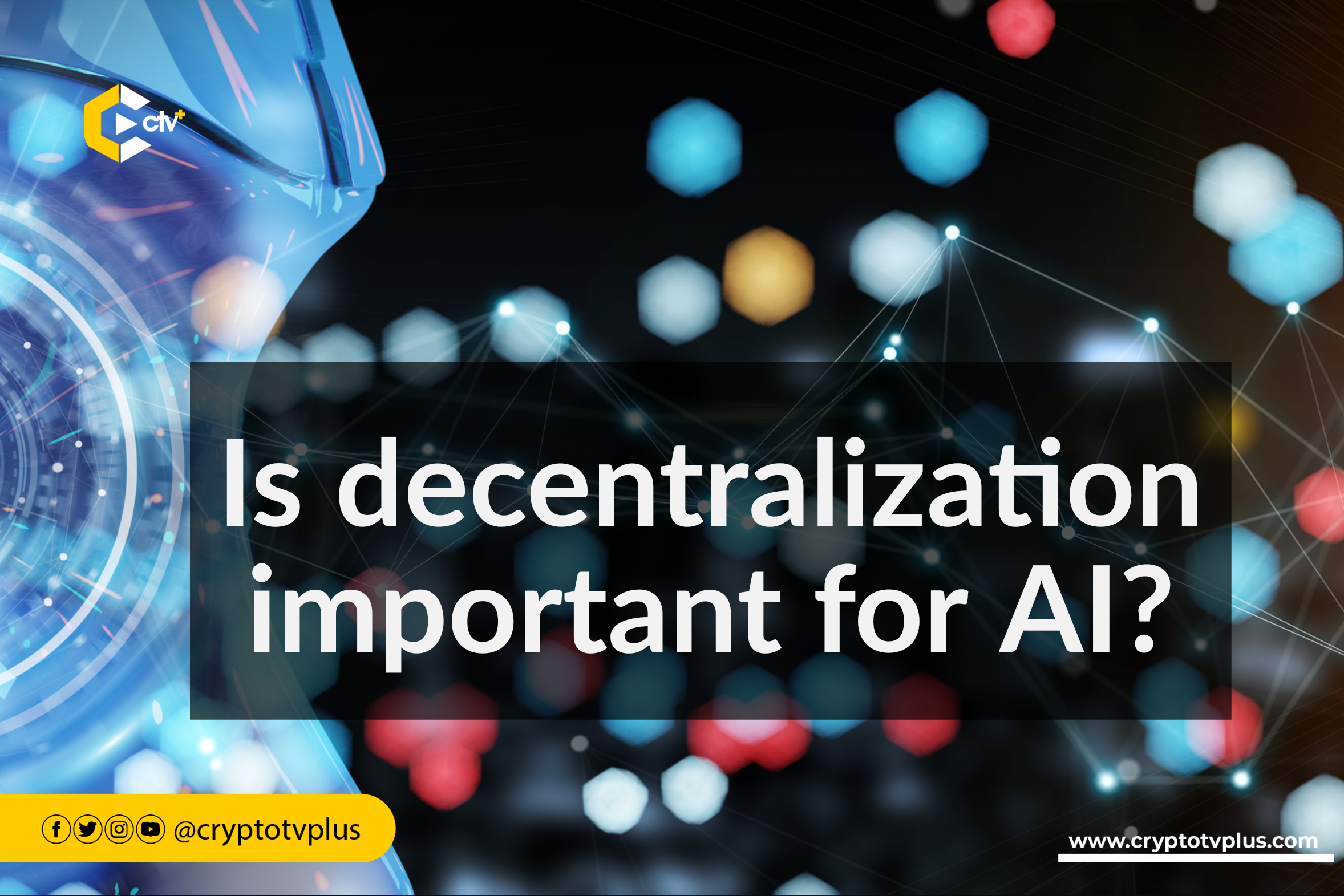
The rise of AI is revolutionizing entire industries and global economies. Companies like Amazon are leveraging robotics in their supply chains, while content creators are utilizing powerful tools like ChatGPT. This signifies a new era of technological progress that we are currently experiencing.
Within the realm of AI development and infrastructure enhancement, industry experts engage in ongoing debates regarding the underlying foundations of AI models.
One key area of focus revolves around incorporating decentralization into these models, even in the presence of corporate entities like OpenAI and others.
At the recently concluded event organized by the Berkeley Center for Responsible, Decentralized Intelligence, UC Berkeley, Tatsunori Hashimoto, Assistant Professor, Stanford University, said during a panelist session that decentralization has an important role to play in the AI world.
Reflecting on how legacy internet platforms such as Wikipedia have thrived over the years as a good source of information, he said that the same success can be realized in the world of AI if decentralization comes in.
Another project he mentioned is OpenStreetMap. OpenStreetMap (OSM) is a global, open-source mapping project where individuals and organizations collaborate to create and update a free, editable map of the world.
It offers free and open geographic data, is widely used in various applications, and relies on a community-driven approach for data collection and maintenance.
For Tatsunori, the two projects have shown how communities can collaborate to build data. Conversely, he noted that this approach has not been as prevalent in the development of large language models.
Decentralization and AI for science
Bo Li, who is an Associate Professor at the University of Chicago, talked about the importance of algorithms as an additional perspective in the discussion about decentralization.
He added that open source is important in science as it is useful for the collection of data from open communities, which is harder in closed proprietary settings.
Bo noted that as different types of algorithms are emerging from different sources, including large projects, promoting AI for Science, engagement with open source communities will foster innovation in diverse perspectives.
Open Source vs. Closed Source: The challenges and future ahead
Further into the conversation, Tatsunori said that while corporations are focused on executing specific projects, the open source community explores various data generation methods, improving the models, and integrating them into tools.
He noted this approach allows for the discovery of undiscovered technologies that could potentially surpass proprietary systems.
He also believes that the future will consist of larger communities coming together to build AI systems, especially considering that data can be generated by anyone.
Again, Ion Stoica, Professor of Computer Science, University of California, Berkeley, pointed out that the success of open source technology depends on three critical factors: data, people, and resources.
While many researchers are working on these challenges, open source companies like OpenAI currently have an edge in terms of data quality and resources.
He hinted that despite the challenge, progress is being made in improving data quality and resource efficiency. But although there is progress made in terms of resources and talent, Nazneen Rajani, Research Lead at Hugging Face said, the gap between closed and open source technology may start widening again, despite progress in terms of resources and talent.
She revealed that undisclosed nature of the GPT-4 model is an example of how proprietary companies can affect the future of AI development in terms of how open or close they will be.
Read also; Open source in machine learning: experts weigh in on the future
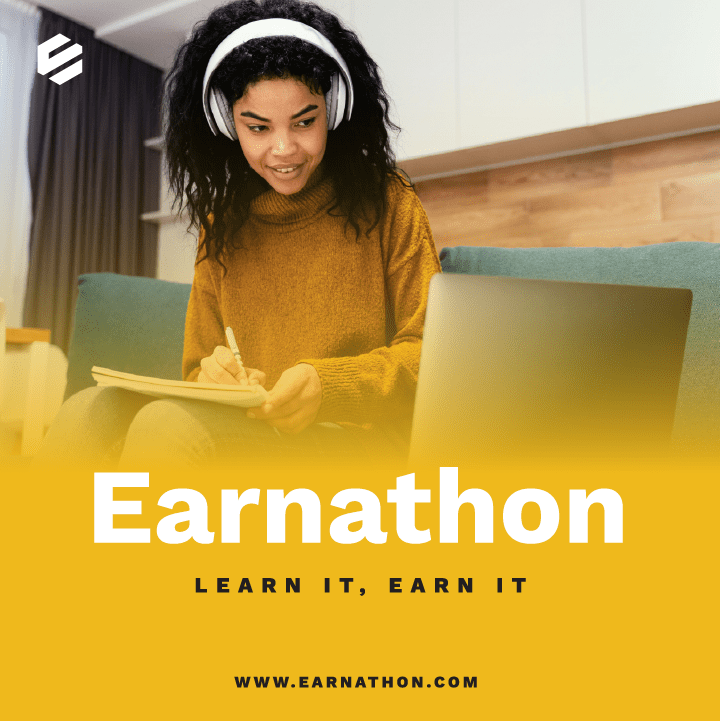